Decentralized AI: Reinventing Cybersecurity for a New Era
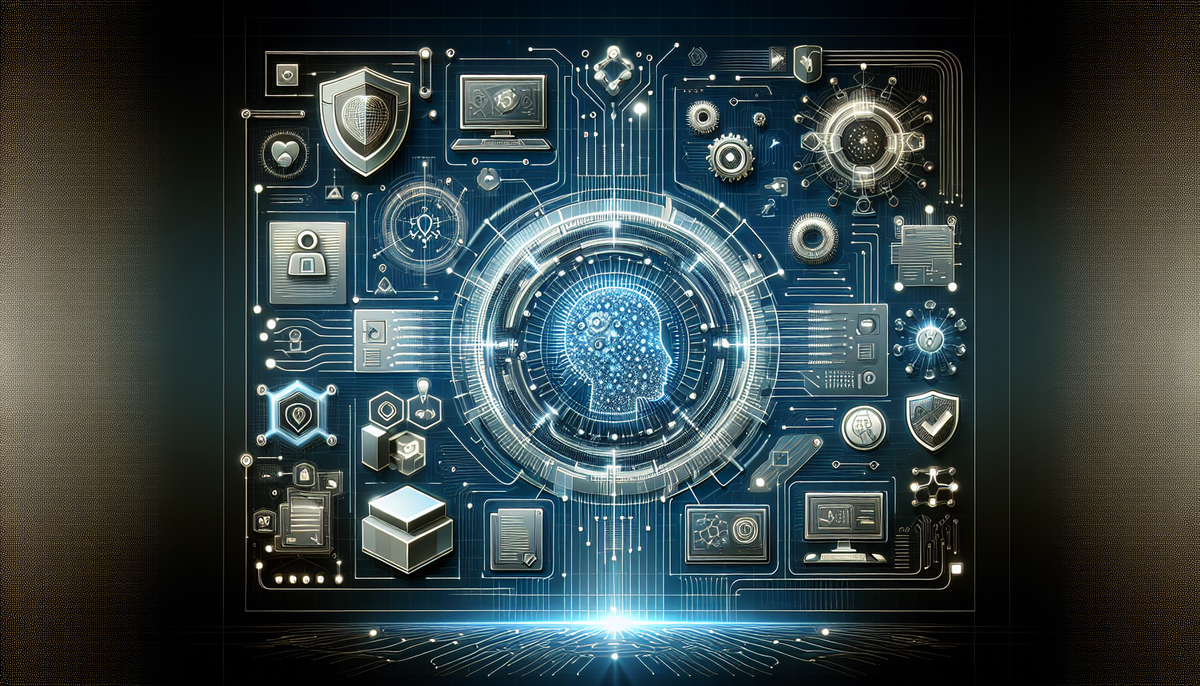
Introduction: A New Era of Cyber Defense
The technology landscape is evolving at an unprecedented pace, driven by trends such as decentralization and artificial intelligence. One of the most promising innovations is decentralized AI, a breakthrough that is not only revolutionizing data processing but also reinventing cybersecurity models. Researchers from IEEE and MIT Tech Review are highlighting that the integration of decentralized AI into security infrastructures can significantly enhance digital defenses, reduce vulnerabilities, and create resilient networks.
The Emergence of Decentralized AI in Cybersecurity
Traditional cybersecurity approaches have largely relied on centralized models for data management and threat detection. However, these systems are increasingly exposed to risks such as single points of failure and coordinated attacks. Decentralized AI offers a distributed method to analyze data across multiple nodes, creating a robust, self-healing security mechanism that can adapt to emerging threats in real time.
How Decentralized AI Works
Decentralized AI leverages advanced algorithms and machine learning frameworks that operate across a network of independent devices. Instead of funneling all data through a central system, each node contributes to the broader analysis, ensuring that any potential breach in one area does not compromise the entire network.
- Distributed Data Analysis: Each unit processes local data and shares insights with the network, ensuring quicker isolation of threats.
- Enhanced Resilience: With no single point of failure, decentralized systems are far more robust against targeted attacks.
- Real-Time Response: Nodes continuously learn from new data, enabling immediate adjustments to evolving threats.
Advantages Over Centralized Systems
The adoption of decentralized AI in cybersecurity brings several important benefits. Here are some key advantages:
- Increased Fault Tolerance: Even if one node is compromised, the overall network remains intact and operational.
- Scalability: As the number of nodes increases, so does the system's ability to process larger volumes of data without performance degradation.
- Efficient Resource Allocation: With distributed processing, computational loads are balanced across the network, optimizing performance.
- Adaptive Security Measures: Continuous learning algorithms enable the system to adapt quickly to new threats, often outpacing traditional static defenses.
The Science Behind the Innovation
At the heart of decentralized AI is the ability to mimic human-like cognitive functions through neural networks that distribute processing tasks. This approach is inspired by natural processes and human brain functioning where decisions are made cooperatively among billions of neurons. Recent research published on Arxiv and papers by NIST have further detailed how these networks can be used for cybersecurity to detect anomalies and predict potential breaches before they impact the system.
Industry Impact and Real-World Applications
Several leading companies and research institutions are already integrating decentralized AI into their cybersecurity protocols. For instance:
- Financial Institutions: Banks and fintech companies are employing decentralized AI to monitor transactions and detect fraudulent activity in real time.
- Healthcare Systems: Hospitals use these technologies to safeguard patient data and ensure compliance with regulatory standards.
- Public Infrastructure: Government agencies are beginning to adopt decentralized frameworks to protect critical infrastructure against cyberterrorism.
Case Study: Revolutionizing Network Security
An international consortium of tech companies recently conducted a study to evaluate the effectiveness of decentralized AI in mitigating cyber threats. The trial involved deploying AI nodes across a simulated network to monitor for intrusions and breaches. The results were groundbreaking:
- Rapid Threat Identification: The AI system detected anomalies 40% faster compared to traditional centralized firewalls.
- Resilience Under Attack: Even when multiple nodes were attacked simultaneously, the network continued to operate with minimal disruptions.
- Cost Efficiency: The distributed model required lower maintenance costs due to its autonomous self-regulating capabilities.
Challenges and Considerations
While decentralized AI offers numerous advantages, it is not without challenges. Experts caution that:
- Integration Complexity: Migrating from centralized systems to a distributed architecture involves significant technical challenges and upfront investment.
- Data Privacy: Ensuring that data remains secure while being processed across multiple nodes requires robust encryption methods and strict regulatory compliance.
- Standardization: As a relatively new technology, decentralized AI lacks the standardized protocols found in more traditional cybersecurity methodologies.
Expert Opinions and Future Outlook
Many cybersecurity experts are optimistic about the transformative potential of decentralized AI. Dr. Elena Martinez, a cybersecurity analyst at MIT Tech Review, commented, "Decentralized AI is poised to create security systems that not only predict and prevent breaches but adapt in real time. The future of digital defense lies in our ability to innovate beyond traditional, centralized paradigms."
Furthermore, leading research institutions are investing heavily in this field. IEEE recently hosted a symposium where experts from across the globe discussed the evolution of decentralized networks and their implications for maintaining privacy and security in an increasingly digital world.
Technical Deep Dive: How It All Comes Together
From a technical perspective, decentralized AI systems rely on several key components:
- Edge AI Processing: By processing data at the edge of the network, these systems reduce latency and improve response times.
- Blockchain Integration: Some systems incorporate blockchain to ensure that data integrity is maintained across decentralized nodes.
- Post-Quantum Cryptography: As cyber threats evolve, researchers are turning to post-quantum cryptography to secure decentralized networks against future attacks.
Implementing Decentralized AI in Your Organization
For businesses interested in leveraging decentralized AI for enhanced cybersecurity, here are actionable steps based on expert recommendations:
- Assess Your Current Infrastructure: Evaluate the strengths and weaknesses of your existing cybersecurity systems to identify areas where decentralization could have the most impact.
- Invest in Research and Development: Partner with technology providers that specialize in AI-driven security solutions and allocate resources for pilot projects.
- Develop a Phased Transition Plan: Transition to a decentralized architecture gradually to ensure continuity, starting with non-critical systems before scaling up.
- Prioritize Compliance and Privacy: Consult with legal experts to ensure all data processing meets regulatory standards such as GDPR and HIPAA.
- Monitor and Adapt: Utilize real-time analytics tools to constantly monitor the performance of decentralized nodes and refine strategies accordingly.
Comparative Analysis: Centralized vs. Decentralized AI
To further understand the benefits of decentralized AI in the realm of cybersecurity, consider the following comparison:
Feature | Centralized AI | Decentralized AI |
---|---|---|
Single Point of Failure | High risk | Minimized risk |
Scalability | Limited by central server capacity | Easily scaled across multiple nodes |
Response Time | Can be slower during peak loads | Rapid response due to distributed processing |
Data Privacy | Concentrated risk if breached | Enhanced through local processing and encryption |
Research and Development: Shaping the Future
Academic and industry research is continually pushing the boundaries of what decentralized AI can achieve. Notable contributions include:
- A recent study from Arxiv detailing how decentralized networks reduce the risk of data tampering and leakage.
- Whitepapers from tech giants like IBM and Intel, which explore the integration of neuromorphic computing with decentralized frameworks.
- Collaborative research initiatives led by NIST, focusing on developing security protocols that can operate efficiently in a decentralized environment.
Potential Challenges and How to Overcome Them
Despite its potential, the transition to decentralized AI is not without hurdles. Key challenges include:
- Integration Complexity: Seamlessly linking legacy systems with new decentralized modules requires robust planning and technical expertise.
- Data Synchronization: Maintaining consistency across distributed databases can be challenging, necessitating innovative synchronization protocols.
- Cybersecurity Regulations: Constantly evolving regulations require that decentralized AI systems remain flexible and compliant at all times.
To address these challenges, experts recommend investing in robust middleware solutions and collaborating with regulatory bodies during system design and implementation.
Expert Insights: Key Takeaways
In summary, the integration of decentralized AI into cybersecurity infrastructure offers transformative benefits by:
- Eliminating single points of failure through distributed risk management.
- Enhancing real-time threat detection and response capabilities.
- Enabling scalable, cost-effective security solutions across diverse industries.
- Incorporating innovative technologies such as blockchain and post-quantum cryptography to future-proof defenses.
As the digital threat landscape continues to evolve, the deployment of decentralized AI will likely become a cornerstone of modern cybersecurity strategies. Stakeholders across industries—from finance to healthcare—must consider these emerging technologies not only as a tool for efficiency but also as a strategic imperative to secure their digital futures.
Conclusion: Embracing Tomorrow's Technology Today
The future of cybersecurity is inherently linked to the evolution of AI. With the increasingly recognized vulnerabilities of centralized systems, decentralized AI offers a promising alternative that combines adaptive learning with resilient architecture. As demonstrated by numerous studies and expert opinions, embracing decentralized AI could lead to a safer, more robust network environment, ensuring that our digital infrastructures are prepared to handle both today’s threats and tomorrow’s challenges.
For organizations looking to secure their networks, the message is clear: proactive innovation is essential. By investing in decentralized AI and integrating it with existing security processes, businesses can create a dynamic defense system that not only reacts to threats but anticipates them. Continuing research from bodies such as IEEE, MIT Tech Review, and NIST reinforces the critical role of decentralized technologies in the future of cybersecurity.
In conclusion, decentralized AI is not just a technological trend, but a fundamental shift in how we perceive and implement digital security. It represents a mature, scalable, and forward-thinking approach that is well-suited to meet the challenges of an increasingly connected world. As organizations worldwide begin to integrate these systems, the promise of a more secure digital future rapidly approaches reality.
To learn more about the technical nuances and industry applications of decentralized AI, readers are encouraged to explore additional resources available from major tech publications and academic journals. The journey to a safer cyber ecosystem is just beginning, and decentralized AI may well be the key to unlocking a new era of digital trust.
Comments ()