Decentralized AI: Shaping The Future of Edge Security
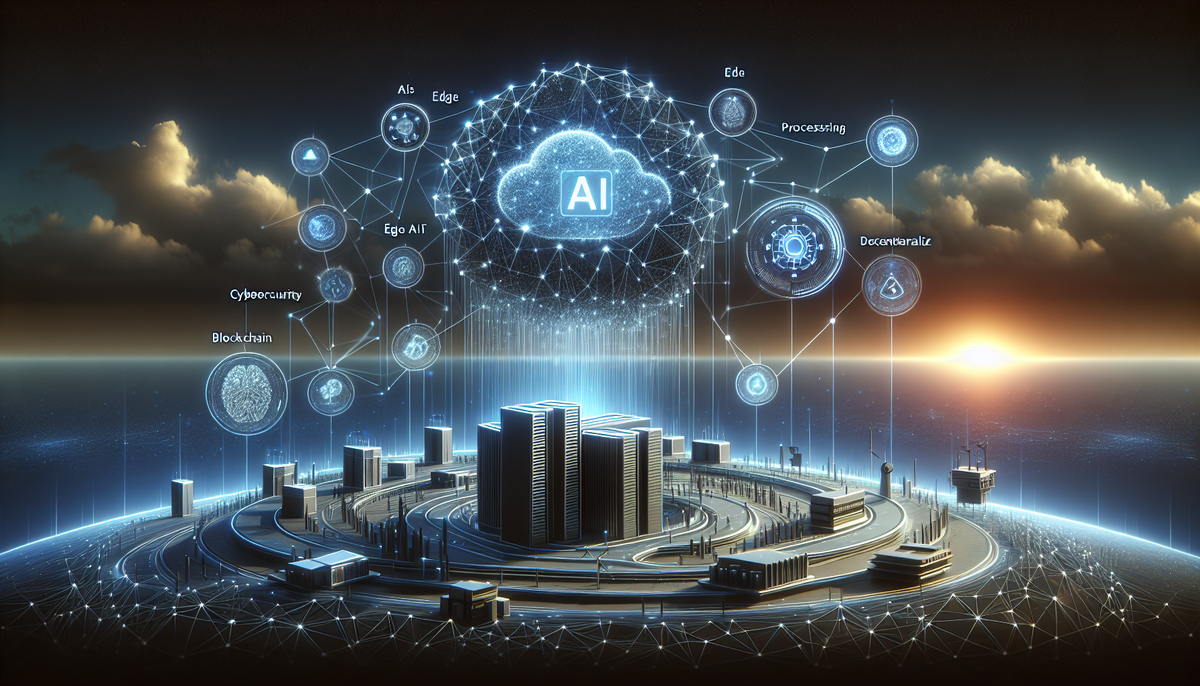
Introduction: A New Era for Cybersecurity
The rapid evolution of artificial intelligence is reshaping every industry, and cybersecurity is no exception. In a world where data breaches and network intrusions are everyday threats, innovative approaches are necessary to secure critical infrastructures. Decentralized AI is emerging as a key player in this mission by distributing decision-making power across a network of devices rather than relying on centralized control. This shift is not only transforming the methods of cyber defense but also redefining the opportunities for edge processing and blockchain-based security frameworks.
Understanding Decentralized AI in Cyber Defense
At its core, decentralized AI involves deploying intelligent algorithms at the periphery of networks—often referred to as edge devices. By localizing data processing, decentralized models reduce latency, enhance privacy, and create scalable solutions that adapt to rapidly evolving threats. The decentralization approach leverages distributed computing power to enable stronger, more agile cybersecurity measures.
Key aspects include:
- Distributed Data Processing: Rather than sending data to a central server for analysis, edge devices analyze data locally, reducing potential points of failure and data bottlenecks.
- Collaborative Decision-Making: Multiple nodes communicate and collaborate in real time, pooling insights to generate quicker and more efficient responses to threats.
- Enhanced Resilience: In a decentralized framework, a breach in one node does not compromise the entire network, thereby containing potential security incidents more effectively.
Emerging Technologies Driving Decentralized Cybersecurity
Decentralized AI is evolving quickly, fueled by innovations in adjacent technology fields. Among the most notable trends are neuromorphic computing, blockchain-based IoT security, and post-quantum cryptography.
Neuromorphic Computing for Real-Time Analysis
Neuromorphic computing mimics the human brain's neural networks and excels at processing data in a highly energy-efficient manner. When integrated with decentralized AI, neuromorphic chips can provide real-time responses to cyber threats even on low-power edge devices. This breakthrough allows for:
- Instantaneous anomaly detection and threat analysis
- Adaptive learning responses that evolve based on the nature of the attack
- Reduced processing power requirements for robust AI algorithms
Blockchain-Based IoT Security
The rapid proliferation of IoT devices has increased cybersecurity risks as these endpoints often lack robust security protocols. Blockchain technology offers a decentralized, tamper-resistant ledger that can dramatically enhance IoT security by:
- Providing an immutable audit trail for device communications
- Facilitating secure authentication and access control in distributed networks
- Enabling smart contracts to automate security updates and patches
Post-Quantum Cryptography for Future-Ready Security
With quantum computers emerging on the horizon, existing cryptographic protocols face the risk of being rendered obsolete. Post-quantum cryptography aims to develop encryption methods that are resistant to quantum attacks. Integrating these protocols with decentralized AI systems ensures that even as computing power grows, the security frameworks remain unbreakable. The evolution of these algorithms is critical for:
- Shielding sensitive data against future quantum-enabled cyber threats
- Providing a long-term security solution in a constantly evolving threat landscape
- Supporting secure communication channels on edge devices globally
Edge AI Processing: Strengthening the First Line of Defense
Edge AI processing transforms traditional cybersecurity by shifting vital computing tasks to the network’s periphery. This development is increasingly important in environments where data must be processed quickly and securely without reliance on centralized data centers. For instance, in industrial IoT networks and smart city infrastructure, even a brief delay in threat detection can have catastrophic results.
Advantages of edge AI include:
- Latency Reduction: By processing data locally, edge devices can detect and respond to threats almost instantaneously.
- Bandwidth Optimization: Local computing reduces the volume of data that must be transmitted over networks, thereby lowering global bandwidth usage.
- Improved Privacy: Keeping sensitive information on local devices helps to minimize data exposure and enhances user privacy.
Case Study: Transforming Enterprise Cyber Defense
Several forward-thinking organizations are already experimenting with decentralized AI for cybersecurity. Industry leaders are combining edge AI technologies with blockchain-based security protocols to create systems that can monitor, analyze, and counteract threats before they escalate into large-scale breaches.
For example, a multinational financial services firm recently implemented an AI-driven cybersecurity solution that leverages neuromorphic computing at its network edge. By integrating blockchain authentication methods across its IoT network, the firm achieved notable improvements in:
- Threat detection speed
- Reduced downtime in the event of an attack
- Overall system reliability and resilience
The success of this pilot program has encouraged further research and development in decentralized AI, with many experts anticipating its widespread adoption across multiple industries, including healthcare, manufacturing, and government sectors.
Challenges and Considerations
Despite its promising advantages, the implementation of decentralized AI is not without challenges. As with any new technology, there are several hurdles that must be addressed:
- Integration Complexity: Incorporating decentralized systems into legacy infrastructures can be difficult and expensive.
- Standardization Issues: The lack of universally accepted protocols can hinder interoperability between devices manufactured by different vendors.
- Security Risks: Although decentralized models offer improved resilience, they are not entirely immune to sophisticated cyber attacks, particularly if individual nodes are poorly secured.
- Resource Constraints: Deployment in resource-limited environments may require significant adjustments to make the technology viable.
Addressing these challenges will require collaboration among technology companies, academic researchers, and government agencies. Leading research institutions such as MIT Tech Review and IEEE are currently investigating standardization and integration methods to ensure a smoother transition to decentralized network architectures.
Expert Opinions and Industry Insights
Prominent experts in the fields of AI and cybersecurity are optimistic about the future of decentralized AI. Dr. Helena Martinez, a cybersecurity specialist at a renowned research institute, emphasizes that "the shift towards edge-based processing is crucial in mitigating the risks of centralized data breaches. Decentralized AI, when coupled with blockchain and quantum-resistant cryptography, could revolutionize how we defend against increasingly complex cyber threats."
Several recent studies, including those published in Arxiv and supported by data from IEEE, have demonstrated that combining neuromorphic architectures with decentralized algorithms yields superior threat detection performance compared to traditional models. These breakthroughs not only bolster current security protocols but also provide a resilient foundation as the technological landscape evolves.
Looking to the Future: A Roadmap for Innovation
As decentralized AI continues to mature, its potential to reshape cybersecurity becomes ever more evident. Future developments may include:
- The widespread use of AI-generated programming techniques that can autonomously address vulnerabilities and patch security holes in real time.
- Enhanced collaboration among diverse devices, resulting in a global threat intelligence network that transcends individual organizational boundaries.
- Adoption of innovative solutions such as silicon photonics, enabling faster data processing at the edge, further reducing reaction times during cyber attacks.
Industry pioneers are already investing heavily in these areas, suggesting that decentralized AI will play a key role in the next generation of cybersecurity defenses. By harnessing the combined power of edge AI processing and advanced cryptographic methods, businesses and governments alike can establish a more secure digital ecosystem that is robust against both current and future threats.
Conclusion: Decentralized AI as a Catalyst for Change
The future of cybersecurity lies in the integration of emerging technologies that challenge traditional models of defense. Decentralized AI represents not simply a new tool in the cyber arsenal, but a fundamental shift towards a more distributed, resilient, and intelligent approach. With advancements in neuromorphic computing, blockchain-based IoT security, and post-quantum cryptography, we are on the verge of a transformational era where our digital infrastructures are defended by systems that are as agile and adaptive as the threats they counter.
As the technology matures, organizations that successfully integrate these cutting-edge solutions will enjoy significant competitive advantages, enhanced data protection, and a proactive posture against cyber threats. For further insights on this evolving landscape, consult recent publications from MIT Tech Review, IEEE, and Arxiv that delve into these innovations. The decentralization of AI not only promises to secure edge networks but also offers a glimpse into a more secure and interconnected digital future.
This article has explored the multifaceted impact of decentralized AI on cybersecurity. As we venture further into the era of edge computing, the challenges will be substantial, yet the rewards—a robust, agile, and highly secure environment—are well within reach. This new frontier is setting the stage for a future where digital defenses are smarter, faster, and ultimately, more reliable.
By embracing these technologies and encouraging collaboration across research, technology, and regulatory domains, we can ensure that the transformative potential of decentralized AI is fully realized. The journey ahead will be complex, but with the continuous support of pioneering research and innovative industry practices, the future of edge security is brighter than ever.
Comments ()